Purpose | Feature/Attribute | Levels |
---|---|---|
H1a |
Financial transparency | (1) Doesn't engage in transparency; (2) Engages in transparency |
H1b |
Accountability | (1) Doesn't engage in accountability; (2) Engages in accountability |
H2 |
Relationship with host government | (1) Friendly relationship with government; (2) Criticized by government; (3) Under government crackdown |
Comparison |
Organization | (1) Amnesty International; (2) Greenpeace; (3) Oxfam; (4) Red Cross |
Comparison |
Issue area | (1) Emergency response; (2) Environment; (3) Human rights; (4) Refugee relief |
Comparison |
Funding source | (1) Funded primarily by many small private donations; (2) Funded primarily by a handful of wealthy private donors; (3) Funded primarily by government grants |
Why Donors Donate: Disentangling Organizational and Structural Heuristics for International Philanthropy
Non-governmental organizations (NGOs) are a core component of global governance. Over the last two decades, however, many host governments have cracked down on NGOs using a series of laws that adversely affect the availability and use of traditional NGO funding. In response to this dramatic shift in the funding landscape, international NGOs have increasingly turned to individual donors to offset the loss funding. Prior research on NGO fundraising has examined how financial transparency and organizational accountability influence individual donor behavior. How do these elements of private donor decision-making interact with structural-level factors such as worsening host country civic environments when deciding to donate? Using a conjoint experiment with likely donors in the US, we find that transparency and accountability can protect against the dampening effect of host government crackdown and criticism of NGOs. Our results have important implications for how NGOs can adapt to worsening civic environments.
philanthropy, conjoint experiments, donor heuristics, repression, NGOs, civil society, nonprofits
What determines individual donor behavior?
A large body of work in philanthropy explores the factors that shape private donor motivation, including altruism, reputational benefits, and alignment with personal values (Bekkers & Wiepking, 2011; Bilodeau & Slivinski, 1997; Wiepking, 2010). However, this research has overwhelmingly looked at individual donor behavior and motivation for giving to domestic organizations instead of international causes (Bekkers & Wiepking, 2011; Tremblay-Boire & Prakash, 2017). The geographic scope of an organization’s mission matters to donors. Giving to local organizations involves fewer recipients and more observable results, while the benefits of donating to organizations working abroad are less visible and more removed from donors (Casale & Baumann, 2015). In a survey experiment, Tremblay-Boire & Prakash (2017) confirm this, finding that “donors are more likely to donate to a charity operating locally than to a charity providing identical service abroad” (2017, p. 644). This difference in donor preferences for international NGO services has been understudied because conventionally, states and foundations have been among the main funders of INGOs.
The third wave of democratization, the collapse of the Soviet Union, and the spread of a “pro-NGO norm” have led to an increased role and visibility for NGOs in recent decades (Reimann, 2006). Donor states have channeled substantial resources through humanitarian and development NGOs, often in response to donor fears that recipient states would use aid inefficiently (Dietrich, 2013). Institutional donors and private funders perceived NGOs as more efficient, more nimble, less bureaucratic, and more trustworthy than states that face poor governance and weak political institutions (Chaudhry, 2022), and as a result, NGOs have become crucial actors in both development and democratization (Herrold, 2020, pp. 142–143).
But as INGOs became increasingly powerful actors in global policy circles, they faced a number of criticisms. Many organizations struggle with the conflicting imperatives of being large organizations that can operate efficiently at scale, while maintaining the grassroots contacts and consensus building that contributed to their early successes (Bano, 2012; Jalali, 2008; Mitchell et al., 2020; Rahman, 2006). Many nonprofit scandals involve some form of financial wrongdoing (Prakash & Gugerty, 2010). Globally, around 5% of revenue is lost annually due to fraud (Association of Certified Fraud Examiners, 2021). Misappropriation of assets and embezzlement schemes are among the most common forms of financial fraud (Lamothe et al., 2023). These criticisms and concerns, in turn, have led donors to demand greater NGO responsiveness, especially regarding financial transparency and accountability.
Assessing an NGO’s deservingness and efficiency demands substantial time and resources, which individual donors typically lack (Croson & Shang, 2011; Tremblay-Boire & Prakash, 2017). As such, these donors rely on various cues and heuristics when deciding to support an NGO. Nonprofits fundraising from individuals work to shape donor perceptions through the use of framing (Chaudhry & Heiss, 2021). We explore two possible categories of heuristic frames: (1) organization-level heuristics, reflected in NGO practices, and (2) structural heuristics, particularly an NGO’s relationship with its host country. We hypothesize that each type plays a role in shaping individual preferences for engaging in philanthropy.
Organizational heuristics: NGO practices
One powerful heuristic for potential donors is organizational efficacy or the belief that an individual’s donation will make a real difference (Chaudhry & Heiss, 2021). This can manifest itself through multiple mechanisms such as the amount an organization spends on overhead costs, whether it makes its finances public, or whether it participates in voluntary accountability practices, such as listing organizational details on websites like GuideStar and Charity Navigator.
Financial transparency, or the “degree of completeness of information provided by [organizations] to the [public] concerning [their] activities,” (Vaccaro & Madsen, 2006, p. 146) is one tangible heuristic that donors turn to when making decisions decisions. NGOs can engage in financial transparency in a variety of ways such as distribution of audited financial statements and third party intermediaries. Research shows that transparency is associated with greater donations, lower debt, and better governance (Harris et al., 2023; Harris & Neely, 2021; Saxton et al., 2012). It can also enhance donor trust and confidence in organizations (Vaccaro, 2006; Vaccaro & Madsen, 2006).
Previous research has found that media exposés about charity mismanagement can generate negative reputational spillovers for the charitable sector (Gibelman & Gelman, 2004). Diversions, or the unauthorized use of an organization’s assets, including embezzlement and theft can result in a decrease in donations. This effect becomes even stronger with media coverage of diversion (Harris et al., 2023). Organizational financial transparency can reduce actual or perceived information asymmetries between donors and charities, thus potentially increasing an individual’s likelihood of donating to an INGO.
H1a: If NGOs are financially transparent, then individual private donors will have a higher likelihood of supporting or donating to them.
Accountability is another factor that can impact donor willingness to donate, and it is necessary for promoting public trust in NGOs. While transparency is an organization’s willingness to make critical data about the organization public, accountability is the willingness of the organization to explain its action to shareholders (Charity Navigator, 2020). It also encompasses how well an organization’s mission is aligned with its resources and how it manages resources to optimize performance (Sloan, 2009). As NGOs gain more prominence, donors demand more information about their activities and strategies (Tremblay-Boire & Prakash, 2015). Explaining actions to stakeholders can be important because many NGOs—even well-intentioned ones—can devote excessive resources towards marketing and overheads (Bowman, 2006). Private donors, subsequently, tend to avoid NGOs that spend a large share of their revenues for overhead, non-mission-related purposes like administration, management and fundraising. The absence of accountability is associated with decreased public trust, worse reputation, and lower perceived quality (Becker, 2018).
Though individual donors would like to have some assurance that their resources will be used appropriately and organizations are not spending too much on overhead, individuals interested in supporting charities cannot thoroughly vet every aspect of the organization (Chaudhry & Heiss, 2021; Tremblay-Boire & Prakash, 2017). To more easily judge organizational accountability, donors may use ratings by watchdog organizations like Charity Navigator and GuideStar as heuristics to guide their decisions. Research shows that initiatives to address accountability gaps by these organizations can substantially increase donations (Gordon et al., 2009; Sloan, 2009; Tremblay-Boire & Prakash, 2017).
H1b: If NGOs are accountable and hold regular third-party audits, then individual private donors will have a higher likelihood of supporting or donating to them.
Structural heuristics: Host country conditions
While NGOs have control over organizational factors such as transparency and accountability and can work to improve these characteristics and public perception of them, NGOs may have little to no control over structural factors that can also influence philanthropy. These structural factors include political contexts in NGO host countries, especially their environments for civil society organizations. A global cascade of anti-NGO laws in recent decades has created barriers to entry, funding, and advocacy for civic organizations (Bakke et al., 2020; Chaudhry, 2022; K. E. Dupuy et al., 2016; Glasius et al., 2020; Heiss, 2017). As a result, NGOs must spend more time, effort, and resources to ensure their survival—at the expense of pursuing their missions.
Some NGOs have adapted to repressive environments by recreating their organizations and changing their mission to avoid directly confronting the government. Analyzing the impact of anti-NGO laws in Bangladesh and Zambia, scholars have found that when faced with government restrictions, NGOs with broad missions restricted their strategies to service work and abandoned their advocacy work. Those that continued to engage in transnational advocacy changed the targets of their advocacy, the issues they focused on, and the language they used (Fransen et al., 2021). Similarly, in Russia, many NGOs working with foreign partners and lobbying the central government no longer consider it an effective strategy (Sundstrom et al., 2022). Overall, fewer groups may end up focusing on contentious causes, instead focusing on regime-compatible causes.
The changes in civic environments impacting NGO activity have also influenced official donor responses. Scholars have found that while multilateral donors do not reduce aid in response to anti-NGO laws, these laws are associated with a 32% decline in bilateral aid inflows (K. Dupuy & Prakash, 2018). Further, advocacy-oriented donors—i.e., those that fund democracy and civil society promotion activities—reduced their spending by 74%, but did not cut their spending on development projects such as education, health, water, and sanitation (Right et al., 2022). However, previous literature has established that individual private donors do not make the same considerations as official donors when deciding to donate (Chaudhry & Heiss, 2021; Desai & Kharas, 2018). Private foreign aid does not have to deal with the same strategic and political considerations as official aid and therefore, may be in a better position to respond to recipients’ needs on the grounds (Easterly & Williamson, 2011). Anti-NGO repression and civil society laws may act as a heuristic to individuals donors that NGOs undertake crucial work abroad, which is why governments perceive them as threatening and crack down on them. Therefore, restrictions imposed by host-country governments are likely to boost donors’ inclination to donate to affected organizations.
H2: If NGOs face legal crackdowns abroad, then individual private donors will have a higher likelihood of supporting or donating to them.
Examining the joint impact of organizational and structural factors
Identifying the causal link between structural factors and donor behavior is more difficult than measuring the link between organizational factors and donor behavior because (1) NGOs have less direct control over their political environments, and (2) organizational characteristics like financial transparency and accountability can sour the NGO-government relationship—governments may be more likely to target NGOs that lack financial transparency and accountability. Moreover, states pass anti-civil society laws in response to broader political trends within their borders, and they regulate NGOs strategically to maximize the benefits and minimize the costs of working with international NGOs (Heiss, 2017). States are more likely to restrict NGOs when organizations pose a threat to regime stability or preferences—for instance, when INGO issue areas threaten government policies, or when INGOs receive substantial foreign funding (K. E. Dupuy et al., 2015).
Donors show increased willingness to donate to NGOs facing government crackdown/criticism while being financial transparent and accountable. While concerns about NGO mismanagement and lack of financial transparency may signal concerns about an NGO’s operations and its ability to abide by the host country’s regulatory environment, the presence of such transparency and accountability may instead convince donors that government targeting of NGOs may be ill-intentioned rather than simply a manifestation of financial oversight. Donors may view anti-NGO laws and inhospitable civic environments as a sign of governments looking to crack down on groups that seek to keep governments accountable, rather than merely auditing and punishing groups that violate routine regulations.
Therefore, both states and individual donors expand and contract their regulatory environments (for states) and support (for donors) for NGOs in response to the interplay between domestic politics and organizational characteristics. The same NGO feature that can increase the likelihood of government crackdown can also simultaneously serve as a donor heuristic and influence perceptions of NGO deservingness.
Research design
It is possible to study the effects of both organizational and structural heuristics on donor decision making individually, but disentangling the interaction of the two heuristics from individual donor characteristics adds complex dimensionality and makes more standard experimental work difficult, costly, and statistically fraught. To address this, we use conjoint analysis to simultaneously test individual donor preferences in the face of both organizational and structural heuristics. Conjoint analysis is generally applicable whenever individuals face complex, multi-dimensional tradeoffs that make it difficult (or even impossible) to explicitly elicit preferences. Conjoint designs have been used extensively in political science to study complex preferences for elections, laws, public policy, and public opinion (Bansak et al., 2021; Bremer & Bürgisser, 2023; Hainmueller et al., 2014; Kertzer et al., 2021; Mummolo, 2016), and conjoint experiments are becoming more common in nonprofit studies, particularly for studying individual donor behavior (Bachke et al., 2014; Hirschmann et al., 2022; Kottasz, 2004; Silvia et al., 2023). Knudsen & Johannesson (2019) argue that research related to respondent opinions requires conjoint analysis because it accounts for the masking effects of factors as well as the complexity of a respondent’s judgment of credibility. Because we also want to capture the complexity of a respondent’s judgment of philanthropic preferences, we use a conjoint experiment to measure the causal effect of both organizational and structural heuristics on individuals’ preferences for donating to international NGOs.
A conjoint research design also allows us to calculate treatment effects even if respondents do not see every combination of organization attributes, and correspondingly provides a substantial increase in statistical power. In standard factorial experimental designs, participants would ordinarily need to be shown all possible combinations of experimental attributes. In our experiment, we presented respondents with six possible treatments with randomized attributes for each treatment, yielding 576 possible unique combinations of features. Even with respondents answering twelve iterations of the experiment, not every combination was seen. However, as long as all the possible organizational attributes are well randomized and there are no systematic biases toward specific options (i.e. more respondents select the first option because it is the first) or toward earlier iterations of attribute choices (i.e. respondents are more careful and attentive for the first hypothetical organization than the last), we can pool all observations together for specific attributes of interest while marginalizing across all other attributes (Kertzer et al., 2021). This allows us to (1) estimate the effect of each experimental treatment even if some unique combinations of attributes were unseen, and (2) use a much smaller sample size than would be required in a more traditional factorial design.
While the ability to select key attributes and marginalize over others provides us with analytic flexibility, it also raises possible issues with multiple comparisons, p-hacking, and selective cherry-picking (Bansak et al., 2021), especially given the fact that we have 576 possible combinations of independent variables to explore. As such, before launching the survey experiment, we preregistered a subset of confirmatory and exploratory hypotheses at the Open Science Framework. These hypotheses deal specifically with our key research questions about the effect of transparency, accountability, and crackdown on the propensity to donate, along with comparison treatment effects for organization brand name, issue area, and funding sources. We also examine the interaction between transparency, accountability, and host-country relationships. Our preregistration protocol is available at ANONYMIZED_URL
and in the appendix, and our data and reproducible code is available at ANONYMIZED_URL
.1
Sample
We fielded our survey experiment through Centiment, which recruits representative samples of paid (and highly engaged) survey participants online. To see how varying NGO characteristics influence the decision to donate, our sample was representative of the population of people who are likely to donate to charity. We asked potential participants a screening question about their philanthropic behavior early in the survey—if a participant responded that they give once every few years or never, they were disqualified from the study. Our reported causal effects are therefore not representative of the entire population and instead are only valid for people interested in charitable giving. We also included an attention check question early in the survey and removed respondents who failed the question. Importantly, these screening questions were presented prior to the experimental manipulation to avoid post-treatment bias (Montgomery et al., 2018). Following screening, we received 1,016 viable responses. Table A2, Table A3, and Table A4 provide a detailed breakdown of the individual characteristics of our sample. In general, respondents were well balanced across all pre-treatment characteristics, including gender, age, education, income, and attitudes toward charity.
Experimental design
After collecting baseline information on respondent demographics and attitudes toward charity and voluntarism, we showed participants a set of randomly shuffled hypothetical international NGOs described with randomly shuffled features or attributes. In this paper, we hypothesize and empirically test the effect of financial transparency, accountability, and host country relationship on the likelihood of donations. In our experiment, we included a few additional treatments to aid with the interpretation and comparison of effect sizes for our treatments of interest. In total, we varied six different organizational and structural attributes that might have an effect on donor behavior: (1) organization name and branding, (2) organization issue area, (3) financial transparency practices, (4) accountability practices, (5) funding sources, and (6) relationship with host government (see Table 1).
At the time of designing the study, Charity Navigator—a large database of U.S. nonprofit financial information—categorized international nonprofit activities into four general causes: (1) development, (2) humanitarian relief, (3) international affairs and human rights advocacy, and (4) environment.2 Accordingly, we used four nonprofits that are stereotypical for each cause: the International Committee of the Red Cross (humanitarian relief), Amnesty International (human rights), Oxfam (development), and Greenpeace (environment). We also varied several other features, including four issue areas (emergency response, environmental advocacy, human rights advocacy, and refugee relief), two organizational practices (financial transparency and third-party accountability audits), three funding sources (many small private donations, a handful of wealthy private donors, and government grants), and three relationships with host governments (friendly, criticized by the government, and under government crackdown).
After presenting respondents with a set of organizations, we asked them which of the three they would be willing to donate to, along with an option for no selection (see Table 2 for an example). We then repeated the process eleven more times for a total of twelve randomized iterations of hypothetical combinations of attributes, resulting in 12,192 completed experimental tasks (12 iterations × 1,016 respondents).
Attribute | Option 1 | Option 2 | Option 3 | None |
---|---|---|---|---|
Organization | Greenpeace | Oxfam | Red Cross | — |
Issue area | Environment | Refugee relief | Refugee relief | — |
Transparency | Engages in transparency | Doesn't engage in transparency | Doesn't engage in transparency | — |
Accountability | Engages in accountability | Engages in accountability | Engages in accountability | — |
Funding sources | Funded primarily by a handful of wealthy private donors | Funded primarily by government grants | Funded primarily by a handful of wealthy private donors | — |
Relationship with host government | Under government crackdown | Criticized by government | Criticized by government | — |
Modeling and estimands
We analyze the results using a multilevel Bayesian multinomial model.3 Our experimental data has a natural hierarchical structure, with 3 questions nested inside 12 separate experimental tasks, nested inside each of the 1,016 respondents, which lends itself to multilevel modeling. Relying on standard OLS regression can discard valuable information about (1) the relationships and covariance between the different combinations of feature levels offered to respondents, and (2) individual-specific differences in how respondents react to different feature levels (Jensen et al., 2021). Since it was impossible for every respondent to see every possible all 12,000 experimental tasks, multilevel modeling allows us to pool together information about respondents with similar characteristics facing similar sets of choices. Moreover, using random respondent effects provides natural regularization and shrinkage for our estimates—experimental tasks that happened to appear more often due to chance will be accounted for and their frequency will not bias the overall causal effect. We define our model and priors in Equation 1.
\[ \begin{aligned} &\ \mathrlap{\textbf{Multinomial probability of selection of choice}_i \textbf{ in respondent}_j} \\ \text{Choice}_{i_j} \sim&\ \mathrlap{\operatorname{Categorical}(\{\mu_{1,i_j}, \mu_{2,i_j}, \mu_{3,i_j}\})} \\[10pt] &\ \mathrlap{\textbf{Model for probability of each option}} \\ \{\mu_{1,i_j}, \mu_{2,i_j}, \mu_{3,i_j}\} =&\ \mathrlap{\begin{aligned}[t] & (\beta_0 + b_{0_j}) + \beta_{1, 2, 3} \text{Organization}_{i_j} + \beta_{4, 5, 6} \text{Issue area}_{i_j} + \\ &\ \beta_7 \text{Transparency}_{i_j} + \beta_8 \text{Accountability}_{i_j} + \\ &\ \beta_{9, 10} \text{Funding source}_{i_j} + \beta_{11, 12} \text{Government relationship}_{i_j} \end{aligned}}\\[5pt] b_{0_j} \sim&\ \mathcal{N}(0, \sigma_0) && \text{Respondent-specific offsets from global probability}\\[10pt] &\ \textbf{Priors} \\ \beta_{0 \dots 12} \sim&\ \mathcal{N} (0, 3) && \text{Prior for choice-level intercept and coefficients} \\ \sigma_0 \sim&\ \operatorname{Exponential}(1) && \text{Prior for between-respondent variability} \end{aligned} \tag{1}\]
We do not include any respondent-level covariates beyond the treatment variables. Because this is an experimental design, any statistical confounding is accounted for during the process of randomization and covariates should have no systematic effect on treatment effects. We do not work with the raw results of the model directly (though a complete table of results is included in Table A8). Given the conjoint design, we instead create a complete balanced grid of all 576 combinations of feature levels (2 transparency × 2 accountability × 3 government relationships × 4 organizations × 4 issues × 3 funding) and use the model to calculate predicted probabilities of choice selection for each row of possible treatment values. We then collapse this set of predicted probabilities into estimated marginal means (EMMs) for specific features of interested while marginalizing or averaging over all other predicted variables (Bremer & Bürgisser, 2023; Leeper et al., 2020). We report the causal effect of each manipulated feature using the average marginal component effect (AMCE), which is equivalent to the difference in estimated marginal means for specific feature levels. For example, when estimating the marginal means of the transparency treatment, we find the average predicted probability across the 288 rows of the reference grid where transparency is true and across the 288 rows where transparency is false—the difference between these two estimated marginal means is the AMCE, or the causal effect of the treatment holding all other values constant, on the probability scale (see the appendix for an extended illustration and demonstration of the EMM and AMCE calculating process).
Results
We present the posterior distributions of the marginal means and AMCEs for each of our experimental conditions in Figure 1 and provide posterior medians, credible intervals, and other model diagnostics in Table A5 and Table A8. Because AMCEs are relative statements (i.e. contrasts between one feature level and a reference level), we try to use logical reference levels: for binary treatments like transparency and accountability, we calculate the difference between false and true levels; for ordered treatments like host country relationship, we calculate the differences between different levels of crackdown compared to no crackdown. To avoid imposing an artificial order on other unordered treatment variables, we report both marginal means and AMCEs (Leeper et al., 2020).
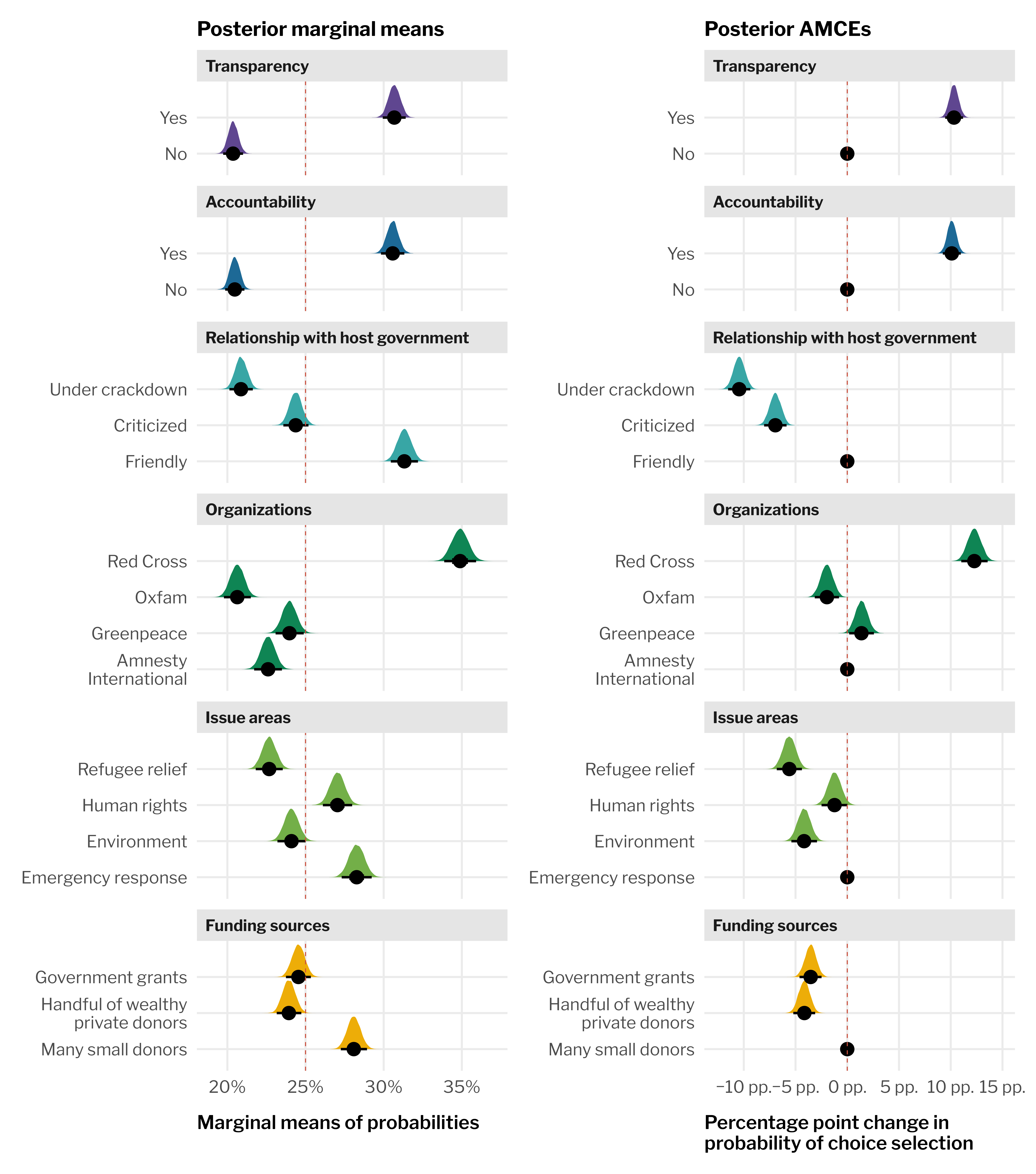
We provide viusalizations of the full posterior distributions of each of the effects of interest, and we report two distributional summary statistics: (1) the posterior median, (2) credible intervals based on a 95% equal-tailed quantile interval, However, we are generally less concerned with the exact point estimates of our causal effects and instead focus on the direction and relative magnitude of their posterior distributions. For inference, we calculate the probability of direction (\(p_d\)), or the probability that the posterior AMCE is strictly positive or negative—it is the proportion of the posterior AMCE that is the sign of the median.
Effect of comparison treatments
To contextualize the magnitude of the causal effects for our hypotheses of interest, we begin our analysis with a brief overview of the effects of organizational characteristics that nonprofits have less direct control over: their brand name, their issue area, and their funding sources. Brand recognition appears to be a powerful heuristic for donor decision making. Respondents were substantially more likely to prefer an organization when it was identified as the Red Cross, with a median posterior marginal mean of 34.9% (95% cred. int. = [0.339, 0.359])—ten percentage points higher than the 25% probability that would be expected if respondents selected an organization at random. When using Amnesty International as the reference category, the AMCE for the Red Cross is 12.3 percentage points (95% cred. int. = [0.110, 0.135]; \(p_d\) = 1.00). Other organizations see much smaller marginal means and AMCEs. Compared to Amnesty International, Greenpeace causes a small 1.4 percentage point increase ([0.002, 0.025]; \(p_d\) = 0.99) and Oxfam causes a small 2.0 percentage point decrease ([−0.031, −0.008]; \(p_d\) = 1.00) in the probability of selecting an organization. The Red Cross brand name heuristic is the strongest of all the experimental treatments and features, signifying the organization’s brand power and goodwill among potential donors.
The issue area an organization works on also serves as a heuristic for donors. As seen in the marginal means in Figure 1, organizations focused on human rights and emergency response are more popular than those working on issues related to the environment or refugee relief. When using emergency response (the most popular issue) as the reference category for AMCEs, working with human rights causes a 1.2 percentage point decrease ([−0.025, 0.000]; \(p_d\) = 0.97), while environmental and refugee relief issues see 4.2 ([−0.054, −0.030]; \(p_d\) = 1.00) and 5.6 ([−0.068, −0.044]; \(p_d\) = 1.00) percentage point decreases, respectively. To put these effects in context, these effects are smaller than the the Red Cross effect and a little larger than the Oxfam and Greenpeace effects.
Finally, an organization’s primary funding source also serves as a reliable donor heuristic. Organizations that are funded by many small donors are substantially more popular than those funded by government grants or a small group of wealthy donors—when compared to many small donors, both government and wealthy individual funding cause 3.5 and 4.2 percentage point decreases in the probability of selection ([−0.046, −0.025]; \(p_d\) = 1.00 and [−0.052, −0.031]; \(p_d\) = 1.00). These effects follow existing research on donor efficacy (Chaudhry & Heiss, 2021)—when donors know that an organization is funded by others like themselves and that the marginal benefit of their individual donation is important, they are more likely to donate. In contrast, when donors know that an organization’s funding does not come from the public and instead comes from a few wealthy donors or large government grants, the marginal benefit they receive from donating decreases and they are less likely to donate. Donors therefore appear to be motivated by some degree of personal efficacy.
Effect of transparency and accountability
Having explored the effects of general organizational characteristics on the propensity to donate, we can test our first hypotheses and examine the effects of our treatments of interest. As seen in the marginal means in Figure 1, both transparency and accountability are strong signals of organizational deservingness. Respondents strongly prefer organizations that engage in either transparency or accountability—both treatments have a posterior marginal mean of roughly 30% compared to a baseline equally-at-random probability of 25% (transparency: 0.307; [0.299, 0.314]; accountability: 0.306; [0.298, 0.313]). The AMCEs for each treatment show a roughly 10 percentage point increase in the probability of selection compared to organizations that do not engage in transparency or accountability (transparency: 0.103; [0.095, 0.112]; \(p_d\) = 1.00; accountability: 0.101; [0.092, 0.110]; \(p_d\) = 1.00). This effect is nearly the same order of magnitude as the Red Cross effect—an NGO that chooses to be more transparent or that takes steps to demonstrate greater accountability can expect to see an increase in the probability of selection equivalent to the boost of the brand name effect associated with the Red Cross. As predicted, we thus find strong support for both H1a and H1b: if NGOs are financially transparent or engage in accountability practices, then individual private donors are roughly 10 percentage points more likely to donate to them. Donors appear to reward NGOs for their efforts to disclose their funding and be more transparent.
There is also some evidence that these two effects are equivalent and perhaps interchangeable. Figure 2 (and Table A6) shows the posterior marginal means for all four combinations of possible levels of transparency and accountability. When respondents see both as “no” simultaneously, the estimated marginal mean is lower than any of the individual treatments in Figure 1, at 16.0% ([0.154, 0.166]), or 9 percentage points lower than the baseline probability of 25%. When either transparency or accountability is set to “yes”, the estimated marginal mean is essentially identical at 30.7% and 30.6%, respectively. When both treatments are set to “yes”, the estimated marginal mean is 36.4% ([0.355, 0.374]), which is roughly the same as the overall estimated marginal mean for the Red Cross. Holding all other treatments constant, the effect of transparency and accountability practices on their own are generally the same, and when combined, the overall favorability of the organization increases substantially. This could indicate that donors do not care about which specific type of organizational practice an NGO engages in and that instead they are looking for some sort of signal that the organization is following best practices in transparency or accountability.
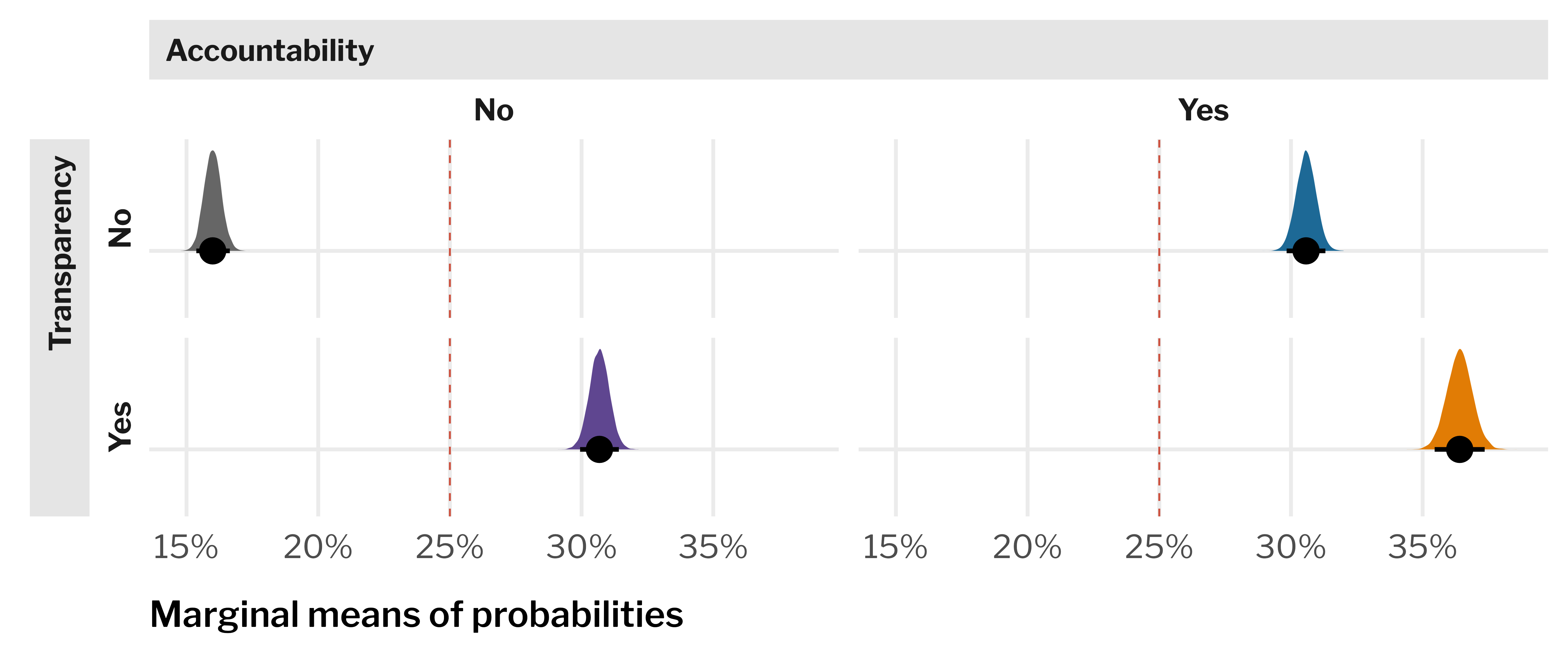
Effect of crackdown
To test our second hypothesis regarding structural heuristics, we explore the effect of an NGO’s host country conditions on the propensity to donate. Contrary to our expectations, respondents appear to prefer donating to NGOs with friendly host government relationships, with a marginal mean of 31.3% (compared to a baseline of 25%; [0.305, 0.322]). Respondents are less likely to donate when an organization is criticized by its host government, and far less likely when an organization faces crackdown. Compared to other treatments, an organization facing crackdown elicits a similar negative preference among potential donors as an organization without transparency or accountability measures. However, an organization with a friendly relationship with the host government leads potential donors to perceive it as equivalent to engaging in transparency or accountability.
In addition to these overall trends in preferences, we can measure the causal effect of moving from friendly NGO–government relations to a more antagonistic relationship. Using friendly relationship as the reference category, facing criticism by the host government causes a 7.0 percentage point reduction in the probability of selection ([−0.080, −0.059]; \(p_d\) = 1.00). Participants respond more strongly as the relationship becomes more conflictual and restricted—an NGO facing crackdown sees a 10.4 percentage point reduction ([−0.115, −0.094]; \(p_d\) = 1.00) in support. We thus find strong evidence against H2: if NGOs face crackdown abroad, individual donors are less likely to donate to them. For context, the causal effect of facing crackdown is the same magnitude as the Red Cross branding effect, but in the opposite direction—donors seem to penalize NGOs facing criticism to the same extent that they reward the Red Cross. This result is surprising, but clarifies findings in previous research. In a similar vignette-based experiment, Chaudhry & Heiss (2021) find no substantial effect of legal restrictions on the probability of donation, and any substantive crackdown-related effects are moderated by other treatments like NGO issue area or funding source. This negative effect might also be related to the notion of donor efficacy. When deciding how to maximize the impact or marginal benefit of their individual donation, donors look for signals that their money will make a difference. Transparency and accountability practices, in addition to knowing that other individuals regularly support the organization, all act as heuristics of efficacy. An NGO facing criticism or crackdown abroad, on the other hand, may signal that potential donations could be used for legal fees, or signal that the NGO had perhaps done something to deserve the legal limitations it faces. Crackdown thus serves as a negative heuristic, signaling that donations might not be used as effectively as donors might hope.
The interaction between transparency, accountability, and crackdown
Financial transparency and accountability practices both have a positive (and likely interchangeable) effect on NGO favorability, while government criticism and crackdown have a negative effect and discourage potential donors from donating. As seen in Figure 3 (and Table A7), these heuristics also interact with each other.4 The estimated posterior marginal means for different relationships with host governments move in the same direction regardless of whether an organization engages in transparency practices: organizations with no conflict are most preferred, while organizations under government crackdown are least preferred. Transparency practices in the presence of crackdown seem to have two general effects. First, engaging in transparency offsets most of the negative effect of facing crackdown. The estimated marginal mean for an organization with friendly host government relationships and no transparency is 25.4%, which is equivalent to the estimated marginal mean for an organization under government crackdown that does engage in transparency. On average, donors are indifferent to both situations—again, a marginal mean of 25% represents the probability of selecting an organization at random—but the presence of transparency shifts NGOs facing crackdown from negatively preferred to indifferent, while the absence of transparency shifts NGOs with friendly relationships from positively preferred to indifferent. From a practical perspective, this suggests that NGOs under crackdown can focus on improving specific organizational practices to offset the negative signals that accompany their legal difficulties.
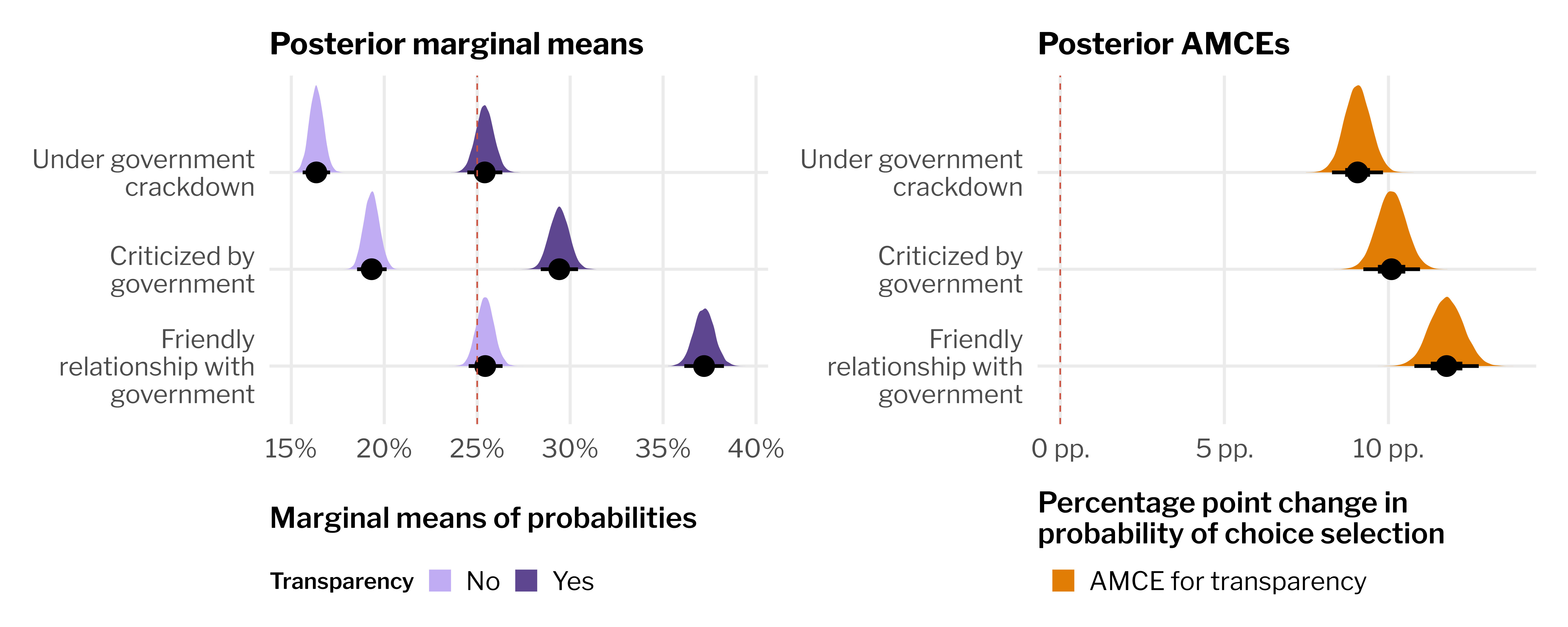
Second, worsening host government relationships weaken the positive effect of transparency. On its own, as seen in Figure 1, engaging in transparency causes a 10.3 percentage point increase in the probability of a respondent selecting an organization. The right panel of Figure 3 (and Table A7) shows how the causal effect of transparency changes across different types of government relationships. These AMCEs represent the difference in the estimated posterior marginal means of the two levels of transparency in the left panel. The effect shrinks as relationships become more negative: under friendly conditions, the median posterior AMCE of transparency is 11.8 percentage points ([0.108, 0.128]; \(p_d\) = 1.00); when an NGO is criticized, the transparency effect is 10.1 percentage points ([0.092, 0.110]; \(p_d\) = 1.00); when an NGO faces crackdown, the effect drops to 9.1 percentage points ([0.083, 0.098]; \(p_d\) = 1.00). The causal effect remains substantially positive regardless of the relationship—even under the worst conditions, engaging in transparency causes a 9 percentage point boost—suggesting that NGOs facing crackdown can still increase their favorability with donors by signalling their commitment to transparency and accountability.
Conclusion
Over recent years, governments globally have systematically controlled and repressed NGOs using both violent tactics and legal means. This trend of closing civic space has important implications for local and international NGOs as well as donors. Recent research has analyzed the impact of this crackdown on public or official aid donors (K. Dupuy & Prakash, 2018; Herrold, 2020; Right et al., 2022). However, we know relatively little about how state repression of NGOs affects the preferences of foreign private donors (Chaudhry & Heiss, 2021). Unlike state donors, individual donors have different motivations for engaging in philanthropy, and they may not necessarily withdraw or reduce support for NGOs facing harassment abroad. This is important because private philanthropy towards organizations working in international affairs continues to grow despite inflation—from 2021 to 2022, individual giving to nonprofits in international affairs grew by over 10.9% in the US (Indiana University Lilly Family School of Philanthropy, 2023).
Given the increasing hurdles faced by these nonprofits, how do individual donors in the U.S. feel about donating to legally besieged NGOs abroad? How do organizational-level factors such as financial transparency and accountability compare with more structural-level factors such as host country civic environments when deciding to donate? Using a conjoint experiment, we demonstrate that organizational and structural characteristics of NGOs serve as cues or heuristics that influence donor preferences. Organizational practices like financial transparency and accountability increase willingness to donate to NGOs. Learning about host government criticism and crackdown against NGOs decreases the likelihood of donation by itself; however, financial transparency and accountability protect against this dampening effect, increasing the probability of philanthropic donations by nine percentage points under the worst conditions of legal crackdown. Our results highlight the importance of organizational characteristics like transparency and accountability even in an era of closing civic space.
These results have important implications for NGO operations, fundraising, and survivability at a time when many INGOs are dealing with hostile host governments. A comparison of the effects of these organizational and structural factors is critical to NGO fundraising—while NGOs have control over transparency and accountability, as well as public perception of these organizational characteristics, they have little to no control over host government crackdowns over their organizations. As a result, they may need to rely entirely on improving individual donor perceptions of organizational transparency and accountability and emphasizing the need for private donor funds at a time of shrinking civic space. This article thus provides insight into the importance of different framing or informational heuristics that can motivate such individual donor giving.
The responses of elite donors and foundations to government crackdown on civil society organizations remains a ripe area for research. Future research should test how well these results map on to elite individual donors and foundations—not just the average private donor. As large foundations are more likely to publish data about their giving than the average private donor, it may be possible to determine how the changes in preferences elicited by these heuristics translate into actual donation behavior. Future work should also test how these findings translate to non-American populations. Due to structural differences in the European nonprofit sector, where NGOs rely less on government funding and more on private funding (Stroup, 2012), donors are likely motivated by different concerns. Government crackdown on civil society organizations is even more challenging for local NGOs in Global South countries, where much government and public support is channeled through service delivery organizations. Domestic NGOs that work on more contentious issues may not be able to raise money locally, as individuals may prefer donating to service organizations focusing on health, education, and poverty (Absar et al., 2017). Moreover, individual donors might also avoid giving to organizations focusing on contentious causes such as advocacy, media freedom, and anti-corruption initiatives due to unfavorable tax benefits or fear of retribution (Baoumi, 2016; Brechenmacher, 2017; K. E. Dupuy et al., 2015). Future research should examine how local NGOs can overcome individual foreign donors’ concerns about host governments’ criticisms of and crackdown on these groups.
Data availability statement
All data and replication code is available at DATAVERSE DOI
and at OSF DOI
.
Supplementary material
A companion statistical analysis notebook with a fuller description of the data, modeling details and diagnostics, and additional explanations of the Bayesian multinomial modeling approach, along with links to the data and replication code, is available at ANONYMIZED URL/DOI
.
Funding
This research received no external funding.
Competing interests
The authors report there are no competing interests to declare.
References
Appendix
Calculating marginal means and AMCEs from a multinomial model using a reference grid
To find the causal effects defined in each of our estimands, we calculate estimated marginal means (EMMs) by finding the fitted probability-scale values for each cell in a balanced reference grid of all 576 possible combinations of feature levels (2 transparency × 2 accountability × 3 government relationships × 4 organizations × 4 issues × 3 funding = 576 rows). We then calculate group averages and contrasts in group averages for each of the features of interest, marginalizing over all other features. At its core, a “marginal mean” refers to the literal mean in the margins in a contingency table of model predictions, and differences in marginal means are equivalent to marginal effects or regression coefficients.
When working with the results for our multinomial regression model, we rely on estimated marginal means rather than raw regression coefficients because of the complexity of the model. As seen in Table A8, the model returns three sets of coefficients per conjoint level. Each coefficient shows the shift in probability that someone will choose an organization from column 1, column 2, or column 3, so we get a coefficient for each of those outcomes (or µ1, µ2, and µ3). Under experimental conditions where cells in the contingency table are randomly assigned, it is safe to assume that the cell proportions are equal and then marginalize (i.e. find the average) across the rows or columns. This allows us to take the average of each set of coefficients (e.g. µ1, µ2, and µ3 for “Transparency = Yes”) to create a single value per coefficient.
To convert EMMs and AMCEs to a more interpretable probability scale (rather than the original log odds scale), we generate predicted values (marginalized across the three µ terms) for each of the 576 unique combinations of feature levels. Table A1 provides an excerpt from this grid, showing six rows where accountability, organization, issue area, and funding are identical and held constant, while transparency and government relations vary.
Organization | Issue | Transparency | Accountability | Funding | Government | EMM |
---|---|---|---|---|---|---|
Red Cross | Emergency response | Transparency: No | Accountability: Yes | Funded primarily by many small private donations | Friendly relationship with government | 0.486 |
Red Cross | Emergency response | Transparency: No | Accountability: Yes | Funded primarily by many small private donations | Criticized by government | 0.396 |
Red Cross | Emergency response | Transparency: No | Accountability: Yes | Funded primarily by many small private donations | Under government crackdown | 0.347 |
Red Cross | Emergency response | Transparency: Yes | Accountability: Yes | Funded primarily by many small private donations | Friendly relationship with government | 0.626 |
Red Cross | Emergency response | Transparency: Yes | Accountability: Yes | Funded primarily by many small private donations | Criticized by government | 0.537 |
Red Cross | Emergency response | Transparency: Yes | Accountability: Yes | Funded primarily by many small private donations | Under government crackdown | 0.485 |
To calculate the marginal mean for a feature, we find the average predicted value across each the levels of that feature. To illustrate, assume that Table A1 represents the full reference grid of all experimental features and levels. The marginal means for transparency would be (0.486 + 0.396 + 0.347)/3 = 0.410 when transparency is set to “no”, and (0.626 + 0.537 + 0.485)/3 = 0.549 when transparency is set to “yes”. In reality, the marginal mean for transparency reported in Figure 1 and Table A5 reflects the average of 288 rows where transparency is no and 288 rows where transparency is yes.
To calculate the AMCE for a feature, we find the difference in estimated marginal means. If we again assume that Table A1 contains the full reference grid, the AMCE for transparency would be 0.549 − 0.410, or 0.140, or 14 percentage points. Again, this is not actually the true causal effect—the real AMCE in Figure 1 and Table A5 is the difference in marginal means for the 288 rows where transparency is no and the 288 rows where transparency is yes.
Additional tables
Demographics | ||
---|---|---|
Response | N | % |
Gender | ||
Male | 517 | 50.9% |
Female | 485 | 47.7% |
Transgender | 8 | 0.8% |
Prefer not to say | 3 | 0.3% |
Other | 3 | 0.3% |
Age | ||
Less than 2017 national median (36) | 179 | 17.6% |
More than median | 837 | 82.4% |
Marital status | ||
Married | 403 | 39.7% |
Widowed | 21 | 2.1% |
Divorced | 104 | 10.2% |
Separated | 35 | 3.4% |
Never married | 453 | 44.6% |
Education | ||
Less than high school | 25 | 2.5% |
High school graduate | 270 | 26.6% |
Some college | 287 | 28.2% |
2 year degree | 138 | 13.6% |
4 year degree | 206 | 20.3% |
Graduate or professional degree | 82 | 8.1% |
Doctorate | 8 | 0.8% |
Income | ||
Less than 2017 national median ($61,372) | 585 | 57.6% |
More than median | 431 | 42.4% |
Attitudes toward charity | ||
---|---|---|
Response | N | % |
Frequency of donating to charity | ||
More than once a month, less than once a year | 566 | 55.7% |
At least once a month | 450 | 44.3% |
Amount of donations to charity last year | ||
$1-$49 | 337 | 33.2% |
$50-$99 | 245 | 24.1% |
$100-$499 | 233 | 22.9% |
$500-$999 | 107 | 10.5% |
$1000-$4,999 | 65 | 6.4% |
$5000-$9,999 | 18 | 1.8% |
$10,000+ | 11 | 1.1% |
Importance of trusting charities | ||
1 (not important) | 7 | 0.7% |
2 | 9 | 0.9% |
3 | 21 | 2.1% |
4 | 98 | 9.6% |
5 | 168 | 16.5% |
6 | 157 | 15.5% |
7 (important) | 556 | 54.7% |
Level of trust in charities | ||
1 (no trust) | 14 | 1.4% |
2 | 20 | 2.0% |
3 | 68 | 6.7% |
4 | 257 | 25.3% |
5 | 328 | 32.3% |
6 | 169 | 16.6% |
7 (complete trust) | 160 | 15.7% |
Frequency of volunteering | ||
Haven't volunteered in past 12 months | 423 | 41.6% |
Rarely | 20 | 2.0% |
More than once a month, less than once a year | 322 | 31.7% |
At least once a month | 251 | 24.7% |
Politics, ideology, and religion | ||
---|---|---|
Response | N | % |
Frequency of following national news | ||
Rarely | 88 | 8.7% |
Once a week | 216 | 21.3% |
At least once a day | 712 | 70.1% |
Traveled to a developing country | ||
Yes | 250 | 24.6% |
No | 766 | 75.4% |
Voted in last election | ||
Yes | 742 | 73.0% |
No | 274 | 27.0% |
Trust in political institutions and the state | ||
1 (no trust) | 123 | 12.1% |
2 | 155 | 15.3% |
3 | 207 | 20.4% |
4 | 276 | 27.2% |
5 | 151 | 14.9% |
6 | 49 | 4.8% |
7 (complete trust) | 55 | 5.4% |
Political ideology | ||
1 (extremely liberal) | 87 | 8.6% |
2 | 87 | 8.6% |
3 | 112 | 11.0% |
4 | 363 | 35.7% |
5 | 175 | 17.2% |
6 | 80 | 7.9% |
7 (extremely conservative) | 112 | 11.0% |
Involvement in activist causes | ||
Not involved | 569 | 56.0% |
Involved | 447 | 44.0% |
Frequency of attending religious services | ||
Not sure | 11 | 1.1% |
Rarely | 600 | 59.1% |
At least once a month | 405 | 39.9% |
Importance of religion | ||
Not important | 338 | 33.3% |
Important | 678 | 66.7% |
Feature | Posterior EMM* | Contrast | Posterior AMCE* | pdirection† |
---|---|---|---|---|
Transparency | ||||
Yes | 0.307 |
Yes−No |
0.103 |
1.00 |
No | 0.204 |
(Reference) |
— | — |
Accountability | ||||
Yes | 0.306 |
Yes−No |
0.101 |
1.00 |
No | 0.205 |
(Reference) |
— | — |
Relationship with host government | ||||
Under government crackdown | 0.209 |
Under government crackdown−Friendly relationship with government |
−0.104 |
1.00 |
Criticized by government | 0.244 |
Criticized by government−Friendly relationship with government |
−0.070 |
1.00 |
Friendly relationship with government | 0.313 |
(Reference) |
— | — |
Organizations | ||||
Red Cross | 0.349 |
Red Cross−Amnesty International |
0.123 |
1.00 |
Oxfam | 0.206 |
Oxfam−Amnesty International |
−0.020 |
1.00 |
Greenpeace | 0.240 |
Greenpeace−Amnesty International |
0.014 |
0.99 |
Amnesty International | 0.226 |
(Reference) |
— | — |
Issue areas | ||||
Refugee relief | 0.227 |
Refugee relief−Emergency response |
−0.056 |
1.00 |
Human rights | 0.270 |
Human rights−Emergency response |
−0.012 |
0.97 |
Environment | 0.241 |
Environment−Emergency response |
−0.042 |
1.00 |
Emergency response | 0.283 |
(Reference) |
— | — |
Funding sources | ||||
Funded primarily by government grants | 0.245 |
Funded primarily by government grants−Funded primarily by many small private donations |
−0.035 |
1.00 |
Funded primarily by a handful of wealthy private donors | 0.239 |
Funded primarily by a handful of wealthy private donors−Funded primarily by many small private donations |
−0.042 |
1.00 |
Funded primarily by many small private donations | 0.281 |
(Reference) |
— | — |
* Values are on the percentage-point scale; single value is posterior median; 95% credible interval in brackets. | ||||
† The probability of direction (pdirection) is the probability that the posterior AMCE is strictly positive or negative—it is the proportion of the posterior AMCE that is the sign of the median. |
Features | Posterior EMM* |
---|---|
Transparency: No | |
Accountability: No | 0.160 |
Accountability: Yes | 0.306 |
Transparency: Yes | |
Accountability: No | 0.307 |
Accountability: Yes | 0.364 |
* Values are on the percentage-point scale; single value is posterior median; 95% credible interval in brackets. |
Feature | Posterior EMM* | Contrast | Posterior ∆* | pdirection† |
---|---|---|---|---|
Relationship with government: Under government crackdown | ||||
Transparency: Yes | 0.254 |
Yes−No |
0.091 |
1.000 |
Transparency: No | 0.163 |
(Reference) |
— | — |
Accountability: Yes | 0.253 |
Yes−No |
0.089 |
1.000 |
Accountability: No | 0.164 |
(Reference) |
— | — |
Relationship with government: Criticized by government | ||||
Transparency: Yes | 0.294 |
Yes−No |
0.101 |
1.000 |
Transparency: No | 0.193 |
(Reference) |
— | — |
Accountability: Yes | 0.293 |
Yes−No |
0.099 |
1.000 |
Accountability: No | 0.194 |
(Reference) |
— | — |
Relationship with government: Friendly relationship with government | ||||
Transparency: Yes | 0.372 |
Yes−No |
0.118 |
1.000 |
Transparency: No | 0.254 |
(Reference) |
— | — |
Accountability: Yes | 0.371 |
Yes−No |
0.115 |
1.000 |
Accountability: No | 0.256 |
(Reference) |
— | — |
* Values are on the percentage-point scale; single value is posterior median; 95% credible interval in brackets. | ||||
† The probability of direction (pdirection) is the probability that the posterior difference in marginal means is strictly positive or negative—it is the proportion of the posterior difference in marginal means that is the sign of the median. |
Feature | Posterior medians | 95% credible intervals | |||||
---|---|---|---|---|---|---|---|
µ1 | µ2 | µ3 | µ1 | µ2 | µ3 | ||
Transparency | Transparency × Yes | 0.52 | 0.61 | 0.59 | [0.45, 0.60] | [0.54, 0.69] | [0.51, 0.67] |
Accountability | Accountability × Yes | 0.53 | 0.60 | 0.56 | [0.45, 0.60] | [0.53, 0.68] | [0.48, 0.64] |
Relationship with host government | Criticized | -0.35 | -0.42 | -0.32 | [-0.44, -0.26] | [-0.51, -0.34] | [-0.41, -0.23] |
Under crackdown | -0.54 | -0.62 | -0.57 | [-0.63, -0.45] | [-0.71, -0.53] | [-0.66, -0.47] | |
Organizations | Greenpeace | 0.162 | 0.097 | -0.024 | [0.053, 0.270] | [-0.011, 0.204] | [-0.134, 0.087] |
Oxfam | -0.061 | -0.136 | -0.166 | [-0.175, 0.051] | [-0.246, -0.025] | [-0.279, -0.054] | |
Red Cross | 0.79 | 0.6 | 0.51 | [0.69, 0.89] | [0.5, 0.7] | [0.41, 0.62] | |
Issue areas | Environment | -0.29 | -0.18 | -0.22 | [-0.39, -0.18] | [-0.28, -0.08] | [-0.33, -0.11] |
Human rights | -0.029 | -0.130 | -0.039 | [-0.127, 0.069] | [-0.232, -0.028] | [-0.145, 0.069] | |
Refugee relief | -0.38 | -0.29 | -0.27 | [-0.48, -0.28] | [-0.39, -0.18] | [-0.38, -0.16] | |
Funding sources | Few wealthy donors | -0.26 | -0.123 | -0.32 | [-0.35, -0.17] | [-0.213, -0.033] | [-0.41, -0.22] |
Government grants | -0.24 | -0.17 | -0.165 | [-0.33, -0.16] | [-0.26, -0.08] | [-0.257, -0.072] | |
Intercept | Intercept | -2.3 | -2.4 | -2.4 | [-2.5, -2.2] | [-2.5, -2.2] | [-2.5, -2.2] |
N | 36576 | 36576 | |||||
Estimates are median posterior log odds from a multinomial logistic regression model with three possible categories, and the columns for µ1, µ2, and µ3 represent estimates for each of the outcomes; |
Preregistration deviations
We made the following deviations from our preregistered protocol (Willroth & Atherton, 2024):
- Type
-
Analysis
- Reason
-
New knowledge
- Timing
-
After results known
- Original wording
-
“We will examine the aggregate marginal posterior distributions of the attribute levels”
- Deviation description
-
This statement was vague and seemed to imply that we would analyze the results of the model by looking only at the raw model coefficients. While is is possible to calculate exact feature contrasts by summing specific combinations of coefficients, we instead calculated estimated marginal means and their contrasts (or AMCEs) using the fitted model.
- Reader impact
-
This deviation should improve readers’ interpretation of the findings, since the reported results are no longer on a log-odds or logit scale, and instead are on a more interpretable percentage point scale—estimated marginal means show the percent of respondents who support an NGO given a specific features, while AMCEs show the percentage point change in support when moving from one feature to another. The risk of bias is minimal as the underlying results are identical whether reported as logit-scale coefficients or marginal means.
- Type
-
Hypotheses
- Reason
-
Stylistic
- Timing
-
After results known
- Original wording
-
Q5a: “Donors will show increased willingness to donate to NGOs that are financially transparent”
- Deviation description
-
We rephrased this as H1a: “If NGOs are financially transparent, then individual private donors will have a higher likelihood of supporting or donating to them.”
- Reader impact
-
This deviation has minimal impact on readers’ interpretation of the findings—it is only rephrased to follow an “if… then…” formulation.
- Type
-
Hypotheses
- Reason
-
New knowledge + stylistic
- Timing
-
Direction restated after data collection, but before results were known; “if… then…” formulation added after results known
- Original wording
-
Q5f: “Donors should be no more or less likely to donate to NGOs that are accountable and hold regular third party audits”
- Deviation description
-
We rephrased this as H1b: “If NGOs are accountable and hold regular third-party audits, then individual private donors will have a higher likelihood of supporting or donating to them.”
- Reader impact
-
This deviation might have some impact on readers’ interpretation of the findings. This deviation was the result of misunderstanding existing work on the effect of nonprofit accountability on donor behavior, and we hypothesized that there would be no effect, contrary to what is predicted by previous research. The risk of bias is low, however—we reversed our prediction after data collection but before we analyzed the data and before the results were known.
- Type
-
Hypotheses
- Reason
-
Stylistic
- Timing
-
After results known
- Original wording
-
Q2a: “Donors will show increased willingness to donate to NGOs that are facing government crackdown or criticism”
- Deviation description
-
We rephrased this as H2: “If NGOs face legal crackdowns abroad, then individual private donors will have a higher likelihood of supporting or donating to them.”
- Reader impact
-
This deviation has minimal impact on readers’ interpretation of the findings—it is only rephrased to follow an “if… then…” formulation.
- Type
-
Hypotheses
- Reason
-
New knowledge
- Timing
-
Accountability prediction added after data collection, but before results were known; “if… then…” formulation added after results known
- Original wording
-
Q5b: “Donors will show increased willingness to donate to NGOs that are criticized by the government/under government crackdown when they are also financially transparent”
- Deviation description
-
We explore the interaction between (1) government crackdown and financial transparency and (2) government crackdown and accountability in the paper, but we only specified the first interaction in the preregistration.
- Reader impact
-
This deviation might have some impact on readers’ interpretation of the findings. The omission of a prediction of the relationship between government crackdown and accountability was inadvertent and we had intended to specify it. The risk of bias is low, as we added the new crackdown+accountability hypothesis after data collection and before the results were known.
- Type
-
Hypotheses
- Reason
-
Narrative
- Timing
-
After data collection, before results were known
- Original wording
-
Q1: Branding; Q3: Issue area; Q4: Funding sources
- Deviation description
-
For the sake of narrative simplicity, we do not explicitly test these three predictions as hypotheses. In this paper, our primary interest is crackdown, transparency, and accountability, but we look at branding, issue area, and funding sources to help compare and give context to the magnitude of our main hypotheses.
- Reader impact
-
This deviation might have some impact on readers’ interpretation of the findings, as it might appear that we have selectively reported a handful of our predictions. To avoid this, and for the sake of full transparency, we include these results in Figure 1 and Table A5. The risk of bias is low—we decided on the narrative framing for this paper after collecting the data but before analyzing the results.
Condensed preregistration
Study information
Title
Why Donors Donate: Disentangling Organizational and Structural Heuristics for International Philanthropy
Research Questions
We use a conjoint survey experiment to examine the impact of organizational features of nongovernmental organizations (NGOs) and the structural factors in target countries in which they operate on donors’ decisions to engage in philanthropy. We explore three research questions in this study:
Do donors rely on structural characteristics of NGOs as heuristics when deciding to donate? How do structural heuristics compare to organizational heuristics?
Donors rely on shortcuts, signals, and heuristics to determine the trustworthiness of NGOs, since seeking out complete information about an organization’s deservingness and efficiency is costly and time-consuming. Previous research has found that an NGO’s organizational characteristics commonly serve as heuristics for donors. Donors use an organization’s overhead costs, the issues it works on, its transparency and accountability practices, and a host of other organizational practices as signals of an organization’s efficiency and deservingness, which then influences their decision to make a donation. These kinds of heuristics are attributes that organizations can typically control—NGOs can publish annual reports, restructure their management, and engage in other strategies to appear more worthy of donation.
Structural characteristics, such as the political and legal environment an NGO faces in its host country, may also serve as signals to donors of NGO deservingness. We are interested in whether the contentiousness of an NGO’s relationship with its host government influences donor decision making. Do donors care if nonprofits they care about are criticized by, persecuted by, or expelled from the countries they work in?
We are also interested in the effect of organizational characteristics on donor decision making. How do managerial practices (financial transparency and accountability systems), funding sources (private donations and government grants), and issue areas (emergency response, environmental issues, human rights, and refugee relief) compare to structural characteristics when deciding to donate? Which heuristics are more influential?
How do individual-level donor characteristics interact with structural and organizational heuristics? Which kinds of people are more or less likely to consider an NGO’s host country political environment, managerial practices, funding sources, or issue area?
The decision to donate to an NGO is not determined solely by an organization’s characteristics. Donors themselves have personality traits, preferences, and experiences that make them more or less likely to engage in philanthropy. We are interested in how individual donor characteristics, such as political ideology, political knowledge, religious attendance, involvement in charitable activities, involvement in activism, and demographic attributes interact with organizational- and structural-level factors.
What is the optimal mix of attribute levels for NGOs interested in maximizing donations?
Finally, given individual donor characteristics and preferences, we are interested in finding the optimal mix of organizational and structural attributes. What might an NGO try to emphasize in its marketing campaigns? Should it highlight its funding sources, managerial practices, issue area, or relationship with its host governments (even if that relationship is negative)?
Hypotheses
For our first set of questions, we predict that:
Branding
- Donors will be more likely to donate to Oxfam and Red Cross compared to Amnesty International and Greenpeace [Mechanism: awareness of need and contentiousness of issue area]
Government crackdown
- Donors will show increased willingness to donate to NGOs that are facing government crackdown or criticism [Mechanism: Governments wouldn’t be cracking down on them if they didn’t perceive a threat from them which means organizations implementing their missions effectively. This perception of efficacy leads to increased donations.]
- Donors will show increased willingness to donate to Oxfam and Red Cross when they are facing government crackdown or criticism compared to when Amnesty or Greenpeace is facing crackdown.
Issue area
- Donors will show increased willingness to donate to NGOs working in less contentious issue areas (emergency response and refugee relief) over more contentious issue areas (environment and human rights)
- Donors will show increased willingness to donate to NGOs facing government crackdown/criticism working in less contentious issue areas (emergency response and refugee relief) over more contentious issue areas (environment and human rights) [Mechanisms: Perceptions of deservingness of NGOs dealing with emergency response and refugee relief. Donors are also more likely to donate to programs that are compatible with government preferences and have easily measurable outputs, which environment and human rights programs often lack. NGOs working on more contentious issue areas may be expelled or shut down, which would be a waste of donor resources, make it less likely that they donate to these groups.]
Funding sources
- Donors will show increased willingness to donate to NGOs that are funded primarily by numerous small private donors compared to NGOs that are funded by a handful of wealhty private donors and government grants [Mechanism: Perception of efficacy - your contribution matters as a small donor. Government funding may also imply lack of independence of government which can reduce the efficiency of an organization.]
- Donors will show increased willingness to donate to NGOs that are facing government crackdown and are funded primarily by numerous small private donors
- Donors will show increased willingness to donate to NGOs that are facing government crackdown and are funded primarily by numerous small private donors and work in less contentious areas (emergency response and refugee relief)
Organizational practices
- Donors will show increased willingness to donate to NGOs that are financially transparent [Mechanism: Perception of efficacy]
- Donors will show increased willingness to donate to NGOs that are criticized by the government/under government crackdown when they are also financially transparent
- Donors will show increased willingness to donate to NGOs that are criticized by the government/under government crackdown when they are also financially transparent and are funded primarily by numerous small private donors
- Donors will show increased willingness to donate to NGOs that are criticized by the government/under government crackdown when they are also financially transparent and work in less contentious areas (emergency response and refugee relief)
- Donors will show increased willingness to donate to NGOs that are criticized by the government/under government crackdown when they are also financially transparent and work in less contentious areas (emergency response and refugee relief) and are funded by numerous small donors
- Donors should be no more or less likely to donate to NGOs that are accountable and hold regular third party audits [Mechanism: Donors don’t necessarily seek assurance through third-party programs/audits and charity watchdogs, but rather through word of mouth, personal scrutiny and local networks]
Because of the nature of our statistical methods, we do not have exact hypotheses for the second and third set of questions. We describe how we answer these questions in the “Follow-up analyses” and “Exploratory analysis” sections below.
Sampling Plan
Existing data
Registration prior to creation of data
Explanation of existing data
We will not use any existing data.
Data collection procedures
Participants will complete a 10-minute survey on Qualtrics. A static version of the survey is accessible at REDACTED
.
Participants of the survey experiment will be recruited through Centiment, a commercial online provider of high quality nonprobability opt-in survey panels. Centiment ensures panel quality by actively recruiting representative samples of the US population and provides monetary incentives and rewards to participants.
To see how varying NGO characteristics influence the decision to donate, our sample will be representative of a population of people who are likely to donate to charity. We ask potential participants a screening question early in the survey (“Q2.5: How often do you donate to charity”). If a participant responds that they give to once every few years or never, they will be disqualified from the study and the survey will end early.
We will provide Centiment with a link to the survey, which is hosted by Qualtrics. Centiment will then distribute the link to their panel. Participants are compensated through Centiment’s internal reward system through cash, points, and other incentives. Centiment does not provide precise details of participant compensation. Centiment states that their compensation is “fair,” and the company’s business model encourages the company to find and maintain high quality panelists. We thus infer that the amount provided is fair and justified. Centiment users receive compensation from the company following the completion of the survey.
Sample size
Our target sample size is 1,000 participants.
Sample size rationale
A sample size of at least 500 respondents is typical for estimating a hierarchical Bayesian model based on conjoint data. We double this amount because we are interested in analyzing subpopulations of respondents, which requires a larger sample, and we had sufficient budget to acquire up to 1,000 respondents.
Stopping rule
Centiment will monitor how many surveys are successfully completed and will solicit responses until our 1,000 target is met.
Design plan
Study type
Experiment: A researcher randomly assigns treatments to study subjects, this includes field or lab experiments. This is also known as an intervention experiment and includes randomized controlled trials.
Blinding
For studies that involve human subjects, they will not know the treatment group to which they have been assigned.
Study design
We use a fractional factorial design. Since no single respondent can possibly see all possible combinations of the attribute levels, we create a number of different versions of the experimental design. We utilize a hierarchical Bayesian model in part to allow for information sharing across like respondents when estimating individual-level preferences for the attribute levels.
Randomization
Every respondent will be randomly assigned a version of the fractional factorial experimental design.
Analysis Plan
Statistical models
We will use a hierarchical Bayesian multinomial logit model with conjugate or otherwise typical priors. The individual-level model is the multinomial logit and the upper-level model of heterogeneity is multivariate normal,
\[ \begin{aligned} \beta &\sim \operatorname{Multivariate} \mathcal{N}(Z \Gamma, \xi) \\ y &\sim \operatorname{Multinomial logit}(X \beta, \varepsilon) \end{aligned} \]
where \(y\) = which alternative the respondent chooses to donate, \(X\) = design matrix of attribute levels, \(\beta\) = latent individual preferences for the attribute levels, \(Z\) = matrix of individual-level covariates, \(\Gamma\) = matrix of coefficients mapping individual-level covariates onto the latent individual-level preferences, and \(\varepsilon\) and \(\xi\) = errors.
Inference criteria
We will examine the aggregate marginal posterior distributions of the attribute levels and use 95% credible intervals to establish “significance.” Effects are “significant” if the 95% credible intervals don’t include 0. Similarly, marginal posterior distributions are “significantly” different if the 95% credible intervals don’t overlap.
We will examine the marginal posterior distributions of the following models:
- Organizational and structural attribute levels with an intercept-only distribution of heterogeneity
- Organizational and structural attribute levels with competing sets of covariates in the distribution of heterogeneity
Finally, we will employ the posterior distribution of model parameters to conduct counterfactual analyses via a market simulator to determine optimal policies.
Data exclusion
We ask potential participants a screening question early in the survey (“Q2.5: How often do you donate to charity”). If a participant responds that they give to once every few years or never, they will be disqualified from the study and the survey will end early.
We include one question (“Q2.11: Please select blue from the following list:”) to monitor respondent attention. In our analysis we will exclude respondents who fail this question.
Missing data
Because all survey questions are required, we do not anticipate issues with incomplete or missing data.
Footnotes
For narrative purposes, we do not explore all our preregistered hypotheses in this study—many of them are nested inside each other (e.g., the effect of branding and issue area and transparency and crackdown simultaneously). We instead focus here on the simplest, non-nested hypotheses. Following Willroth & Atherton (2024), we outline all the deviations from our preregistration protocol in the appendix.↩︎
See http://web.archive.org/web/20170315000746/https://www.charitynavigator.org/index.cfm?bay=search.categories&categoryid=7. Under the explicitly “International” category, Charity Navigator listed Development and Relief Services; International Peace, Security, and Affairs; and Humanitarian Relief Supplies. The “Environment” category is listed separately but contains many INGOs such as Greenpeace and the World Wide Fund for Nature (WWF). At the time of writing, Charity Navigator had changed its taxonomy to include 18 broader categories.↩︎
We use Stan (Stan Development Team, 2023b, p. v2.26.1; 2023a, p. v2.3.1) through R (R Core Team, 2023, p. v4.3.1) and brms (Bürkner, 2017, p. v2.20.5) to estimate the model. We simulate 4 MCMC chains with 5,000 draws in each chain, 1,000 of which are used for warmup, resulting in 16,000 (4,000 × 4) draws per model parameter. We assess convergence with visual inspection, and all chains converge. Complete results from all the models, along with posterior predictive checks, goodness-of-fit measures, and model diagnostics—as well as our code and data—are available
ANONYMIZED_URL
.↩︎We only show the interaction of transparency and crackdown in Figure 3. Given the near interchangeability of the two treatments, the interaction between accountability and crackdown is nearly identical. We include both transparency and accountability in Table A7.↩︎