knitr::opts_chunk$set(cache=FALSE, fig.retina=2,
tidy.opts=list(width.cutoff=120), # For code
options(width=120)) # For output
# Load libraries and data
library(printr)
library(tidyverse)
library(haven)
library(stargazer)
library(xtable)
library(DT)
library(broom)
library(ggstance)
library(forcats)
library(stringr)
library(psych)
source(file.path(PROJHOME, "Analysis", "graphics.R"))
options("xtable.html.table.attributes" = "border=0 class='table table-condensed'")
unlabelled <- function(x) {
if (!haven:::is.labelled(x))
return(x)
attributes(x) <- NULL
x
}
df.raw <- read_sav(file.path(PROJHOME, "Data", "Data_August 2016.sav")) %>%
mutate_each(funs(unlabelled(.))) %>%
mutate(KKKFrame_CivLibs.factor = factor(KKKFrame_CivLibs, levels=0:1,
labels=c("Public safety frame",
"Civil liberties frame")),
GunFrame_CivLibs.factor = factor(GunFrame_CivLibs, levels=0:1,
labels=c("Public safety frame",
"Civil liberties frame"))) %>%
mutate(sex = factor(PPGENDER, levels=c(1, 0), ordered=TRUE,
labels=c("Male", "Female")),
age = factor(ppagect4, levels=1:4, ordered=TRUE,
labels=c("18–29", "30–44", "45–59", "60+")),
race = factor(PPETHM, levels=c(1, 0), ordered=TRUE,
labels=c("White/non-Hispanic", "Other race specified")),
education = factor(PPEDUCAT, levels=1:4, ordered=TRUE,
labels=c("Less than high school", "High school",
"Some college", "Bachelor’s degree or +")))
saveRDS(df.raw, file.path(PROJHOME, "Data", "survey_clean.rds"))
Table 2: Participant demographics
dem.sex <- df.raw %>%
group_by(sex) %>%
summarise(Frequency = n()) %>%
mutate(Percentage = Frequency / sum(Frequency),
`Cumulative %` = cumsum(Percentage)) %>%
mutate(Demographics = as.character(sex)) %>%
select(Demographics, everything(), -sex)
dem.age <- df.raw %>%
group_by(age) %>%
summarise(Frequency = n()) %>%
mutate(Percentage = Frequency / sum(Frequency),
`Cumulative %` = cumsum(Percentage)) %>%
mutate(Demographics = as.character(age)) %>%
select(Demographics, everything(), -age)
dem.race <- df.raw %>%
group_by(race) %>%
summarise(Frequency = n()) %>%
mutate(Percentage = Frequency / sum(Frequency),
`Cumulative %` = cumsum(Percentage)) %>%
mutate(Demographics = as.character(race)) %>%
select(Demographics, everything(), -race)
dem.education <- df.raw %>%
group_by(education) %>%
summarise(Frequency = n()) %>%
mutate(Percentage = Frequency / sum(Frequency),
`Cumulative %` = cumsum(Percentage)) %>%
mutate(Demographics = as.character(education)) %>%
select(Demographics, everything(), -education)
title.row <- function(x) {
data_frame(Demographics = as.character(x), Frequency = NA,
Percentage = NA, `Cumulative %` = NA)
}
dem.full <- bind_rows(title.row("Sex"), dem.sex,
title.row("Age"), dem.age,
title.row("Race/Ethnicity"), dem.race,
title.row("Education"), dem.education) %>%
mutate(Percentage = ifelse(!is.na(Frequency), scales::percent(Percentage), NA),
`Cumulative %` = ifelse(!is.na(Frequency), scales::percent(`Cumulative %`), NA))
pandoc.table(dem.full, justify="lccc")
Sex |
|
|
|
Male |
396 |
48.9% |
48.9% |
Female |
413 |
51.1% |
100.0% |
Age |
|
|
|
18–29 |
123 |
15.2% |
15.2% |
30–44 |
204 |
25.2% |
40.4% |
45–59 |
241 |
29.8% |
70.2% |
60+ |
241 |
29.8% |
100.0% |
Race/Ethnicity |
|
|
|
White/non-Hispanic |
579 |
71.6% |
71.6% |
Other race specified |
230 |
28.4% |
100.0% |
Education |
|
|
|
Less than high school |
97 |
12.0% |
12.0% |
High school |
256 |
31.6% |
43.6% |
Some college |
223 |
27.6% |
71.2% |
Bachelor’s degree or + |
233 |
28.8% |
100.0% |
Table 4: Personality mean scores for entire sample
df.personality.raw <- df.raw %>%
select(OPENNESS, CONSCIENTIOUSNESS, EXTRAVERSION, AGREEABLENESS, NEUROTICISM)
personality.alphas <- psych::alpha(as.data.frame(df.personality.raw),
check.keys=TRUE, warnings=FALSE)$alpha.drop %>%
select(raw_alpha) %>%
mutate(Trait = gsub("-", "", rownames(.)))
df.personality <- df.personality.raw %>%
summarise_all(funs(mean = mean(., na.rm=TRUE),
sd = sd(., na.rm=TRUE),
N = sum(!is.na(.)))) %>%
mutate_all(as.numeric) %>%
gather(key, value) %>%
separate(key, c("Trait", "key"), sep="_") %>%
spread(key, value) %>%
left_join(personality.alphas, by="Trait") %>%
mutate(Trait = str_to_title(Trait)) %>%
mutate(Trait = factor(Trait, levels=c("Openness", "Conscientiousness",
"Extraversion", "Agreeableness",
"Neuroticism"),
ordered=TRUE)) %>%
arrange(Trait) %>%
select(Trait, `Sample mean` = mean, Sd = sd, `Cronbach’s α` = raw_alpha, N)
pandoc.table(df.personality, justify="lcccc", digits=2)
Openness |
0.72 |
0.13 |
0.65 |
760 |
Conscientiousness |
0.78 |
0.13 |
0.61 |
763 |
Extraversion |
0.63 |
0.16 |
0.68 |
755 |
Agreeableness |
0.79 |
0.12 |
0.62 |
753 |
Neuroticism |
0.54 |
0.16 |
0.59 |
771 |
Weighted group means
KKK experiment
Summary statistics
# TODO: Figure out more accurate N; the mean and stdev drop some observations that the N doesn't account for?
kkk.summary <- df.raw %>%
filter(!is.na(KKKFrame_CivLibs.factor)) %>%
group_by(KKKFrame_CivLibs.factor) %>%
summarise(N = n(),
Mean = Hmisc::wtd.mean(KKK_Support, weight, na.rm=TRUE),
`Std. dev.` = sqrt(Hmisc::wtd.var(KKK_Support, weight, na.rm=TRUE)),
`Std. err.` = `Std. dev.` / sqrt(N),
Lower = Mean + (qnorm(0.025) * `Std. err.`),
Upper = Mean + (qnorm(0.975) * `Std. err.`))
kkk.summary
Public safety frame |
273 |
2.665032 |
1.875855 |
0.1135319 |
2.442514 |
2.887551 |
Civil liberties frame |
267 |
3.248471 |
2.165321 |
0.1325155 |
2.988746 |
3.508197 |
One-way ANOVA
kkk.anova <- aov(KKK_Support ~ KKKFrame_CivLibs.factor,
weights=weight, data=df.raw)
kkk.anova %>% xtable %>%
print(type="html")
|
Df
|
Sum Sq
|
Mean Sq
|
F value
|
Pr(>F)
|
KKKFrame_CivLibs.factor
|
1
|
45.62
|
45.62
|
11.09
|
0.0009
|
Residuals
|
532
|
2188.48
|
4.11
|
|
|
Guns experiment
Summary statistics
guns.summary <- df.raw %>%
filter(!is.na(GunFrame_CivLibs.factor)) %>%
group_by(GunFrame_CivLibs.factor) %>%
summarise(N = n(),
Mean = Hmisc::wtd.mean(Gun_Support, weight, na.rm=TRUE),
`Std. dev.` = sqrt(Hmisc::wtd.var(Gun_Support, weight, na.rm=TRUE)),
`Std. err.` = `Std. dev.` / sqrt(N),
Lower = Mean + (qnorm(0.025) * `Std. err.`),
Upper = Mean + (qnorm(0.975) * `Std. err.`))
guns.summary
Public safety frame |
273 |
3.389310 |
2.273794 |
0.1376163 |
3.119587 |
3.659033 |
Civil liberties frame |
269 |
4.215867 |
2.264065 |
0.1380425 |
3.945309 |
4.486425 |
One-way ANOVA
guns.anova <- aov(Gun_Support ~ GunFrame_CivLibs.factor,
weights=weight, data=df.raw)
guns.anova %>% xtable %>%
print(type="html")
|
Df
|
Sum Sq
|
Mean Sq
|
F value
|
Pr(>F)
|
GunFrame_CivLibs.factor
|
1
|
91.31
|
91.31
|
17.72
|
0.0000
|
Residuals
|
532
|
2742.04
|
5.15
|
|
|
Basic OLS models
KKK experiment
model.orig.kkk <- lm(KKK_Support ~ PPETHM + PPGENDER + xparty7 +
KKKFramexO + KKKFramexC + KKKFramexE + KKKFramexA +
KKKFramexN + KKKFrame_CivLibs,
data=df.raw, weights=weight)
model.orig.kkk.interaction <- lm(KKK_Support ~ PPETHM + PPGENDER + xparty7 +
KKKFramexO + KKKFramexC + KKKFramexE +
KKKFramexA + KKKFramexN + KKKFrame_CivLibs +
KKKFramexNC + KKKFramexPK,
data=df.raw, weights=weight)
coef.names.kkk <- names(coef(model.orig.kkk.interaction))
coef.plot.kkk <- bind_rows(mutate(tidy(model.orig.kkk, conf.int=TRUE),
model="Reduced"),
mutate(tidy(model.orig.kkk.interaction, conf.int=TRUE),
model="Full")) %>%
mutate(low = estimate - std.error,
high = estimate + std.error) %>%
left_join(coef.names, by=c("term" = "coef.name")) %>%
mutate(coef.clean = fct_rev(fct_inorder(coef.clean)),
model = factor(model, levels=c("Reduced", "Full"),
labels=c("Reduced ", "Full")))
ggplot(coef.plot.kkk, aes(x=estimate, y=coef.clean, colour=model)) +
geom_vline(xintercept=0, colour="#FF851B") +
geom_pointrangeh(aes(xmin=low, xmax=high), size=0.5,
position=position_dodge(width=0.5)) +
scale_colour_manual(values=framing.palette("palette1"), name=NULL) +
labs(x="Coefficient", y=NULL,
title="KKK framing experiment",
subtitle="Reduced and full models") +
theme_framing(12) + theme(legend.position="bottom")
## Warning: position_dodge requires non-overlapping x intervals
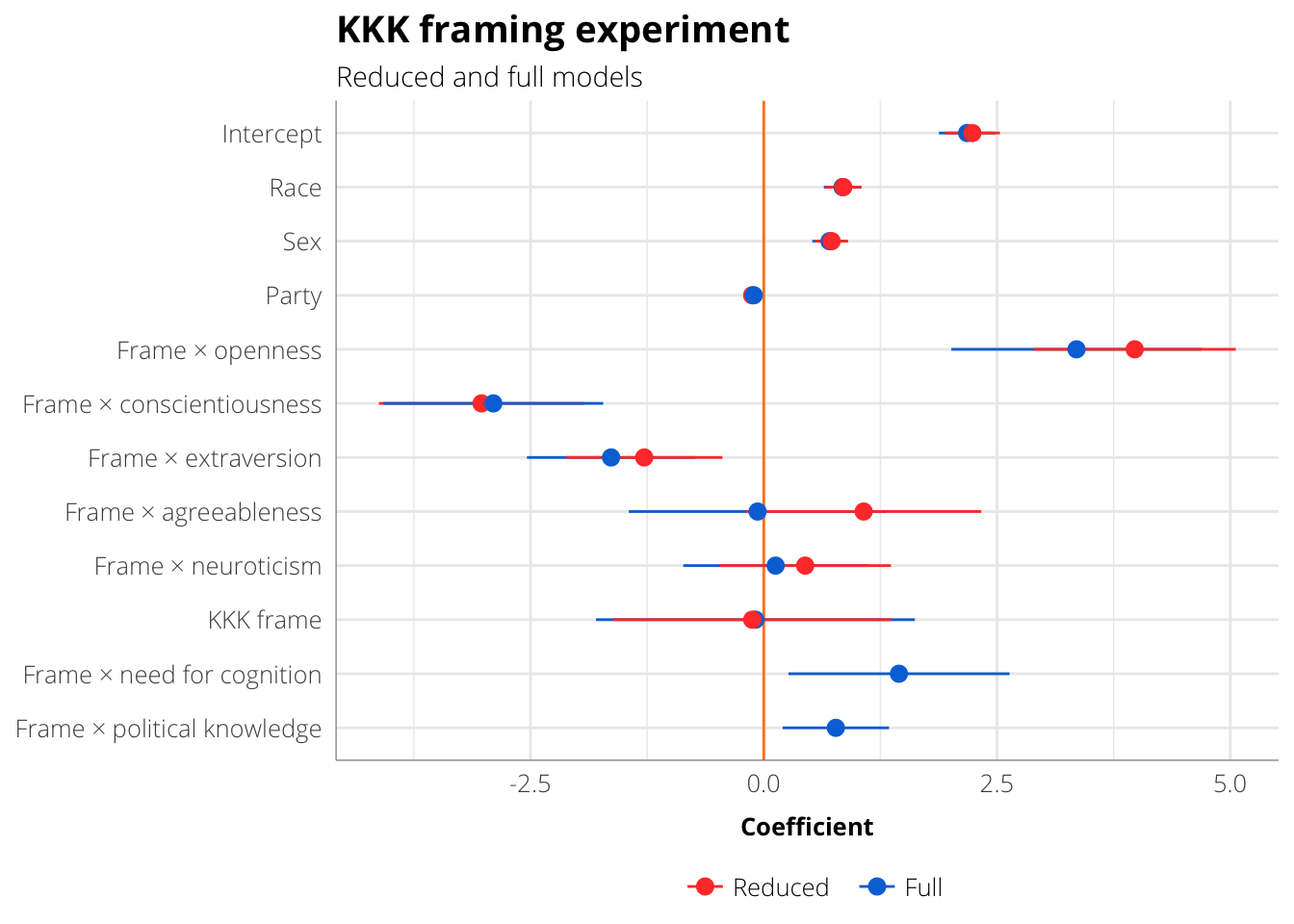
stargazer(model.orig.kkk, model.orig.kkk.interaction,
type="html", intercept.bottom=FALSE,
dep.var.caption="", dep.var.labels.include=FALSE,
report="vcsp*")
|
|
(1)
|
(2)
|
|
Constant
|
2.236
|
2.180
|
|
(0.295)
|
(0.303)
|
|
p = 0.000***
|
p = 0.000***
|
|
|
|
PPETHM
|
0.853
|
0.844
|
|
(0.195)
|
(0.200)
|
|
p = 0.00002***
|
p = 0.00003***
|
|
|
|
PPGENDER
|
0.727
|
0.704
|
|
(0.176)
|
(0.184)
|
|
p = 0.00005***
|
p = 0.0002***
|
|
|
|
xparty7
|
-0.124
|
-0.107
|
|
(0.043)
|
(0.044)
|
|
p = 0.004***
|
p = 0.017**
|
|
|
|
KKKFramexO
|
3.975
|
3.351
|
|
(1.083)
|
(1.342)
|
|
p = 0.0003***
|
p = 0.013**
|
|
|
|
KKKFramexC
|
-3.025
|
-2.898
|
|
(1.100)
|
(1.177)
|
|
p = 0.007***
|
p = 0.015**
|
|
|
|
KKKFramexE
|
-1.281
|
-1.636
|
|
(0.839)
|
(0.902)
|
|
p = 0.128
|
p = 0.071*
|
|
|
|
KKKFramexA
|
1.070
|
-0.066
|
|
(1.261)
|
(1.380)
|
|
p = 0.397
|
p = 0.962
|
|
|
|
KKKFramexN
|
0.444
|
0.127
|
|
(0.919)
|
(0.989)
|
|
p = 0.630
|
p = 0.898
|
|
|
|
KKKFrame_CivLibs
|
-0.123
|
-0.090
|
|
(1.492)
|
(1.708)
|
|
p = 0.935
|
p = 0.959
|
|
|
|
KKKFramexNC
|
|
1.449
|
|
|
(1.185)
|
|
|
p = 0.223
|
|
|
|
KKKFramexPK
|
|
0.772
|
|
|
(0.570)
|
|
|
p = 0.177
|
|
|
|
|
Observations
|
481
|
456
|
R2
|
0.183
|
0.200
|
Adjusted R2
|
0.167
|
0.181
|
Residual Std. Error
|
1.864 (df = 471)
|
1.870 (df = 444)
|
F Statistic
|
11.729*** (df = 9; 471)
|
10.116*** (df = 11; 444)
|
|
Note:
|
p<0.1; p<0.05; p<0.01
|
Guns experiment
model.orig.guns <- lm(Gun_Support ~ GunFrame_CivLibs + PPETHM + PPGENDER +
xparty7 + GunFramexN + GunFramexO +
GunFramexC + GunFramexE + GunFramexA,
data=df.raw, weights=weight)
model.orig.guns.interaction <- lm(Gun_Support ~ GunFrame_CivLibs + PPETHM +
PPGENDER + xparty7 + GunFramexN +
GunFramexO + GunFramexC + GunFramexE +
GunFramexA + GunFramexPK + GunFramexNC,
data=df.raw, weights=weight)
coef.names.guns <- names(coef(model.orig.guns.interaction))
coef.plot.guns <- bind_rows(mutate(tidy(model.orig.guns, conf.int=TRUE),
model="Reduced"),
mutate(tidy(model.orig.guns.interaction, conf.int=TRUE),
model="Full")) %>%
mutate(low = estimate - std.error,
high = estimate + std.error) %>%
left_join(coef.names, by=c("term" = "coef.name")) %>%
mutate(coef.clean = fct_rev(fct_inorder(coef.clean)),
model = factor(model, levels=c("Reduced", "Full"),
labels=c("Reduced ", "Full")))
ggplot(coef.plot.guns, aes(x=estimate, y=coef.clean, colour=model)) +
geom_vline(xintercept=0, colour="#FF851B") +
geom_pointrangeh(aes(xmin=low, xmax=high), size=0.5,
position=position_dodge(width=0.5)) +
scale_colour_manual(values=framing.palette("palette1"), name=NULL) +
labs(x="Coefficient", y=NULL,
title="Concealed carry framing experiment",
subtitle="Reduced and full models") +
theme_framing(12) + theme(legend.position="bottom")
## Warning: position_dodge requires non-overlapping x intervals
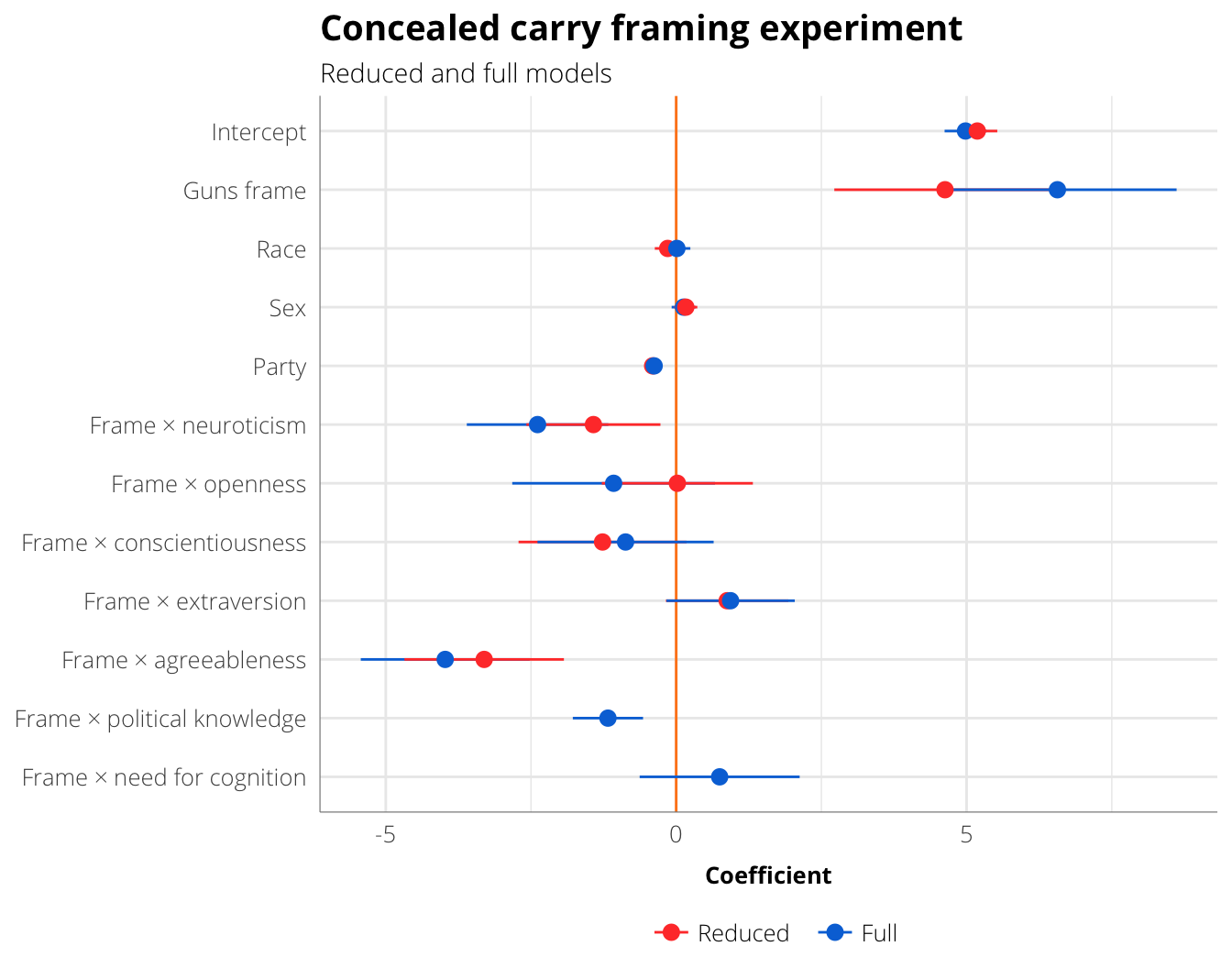
stargazer(model.orig.guns, model.orig.guns.interaction,
type="html", intercept.bottom=FALSE,
dep.var.caption="", dep.var.labels.include=FALSE,
report="vcsp*")
|
|
(1)
|
(2)
|
|
Constant
|
5.184
|
4.981
|
|
(0.345)
|
(0.360)
|
|
p = 0.000***
|
p = 0.000***
|
|
|
|
GunFrame_CivLibs
|
4.629
|
6.564
|
|
(1.906)
|
(2.052)
|
|
p = 0.016**
|
p = 0.002***
|
|
|
|
PPETHM
|
-0.146
|
0.012
|
|
(0.222)
|
(0.232)
|
|
p = 0.510
|
p = 0.960
|
|
|
|
PPGENDER
|
0.166
|
0.126
|
|
(0.197)
|
(0.205)
|
|
p = 0.401
|
p = 0.537
|
|
|
|
xparty7
|
-0.406
|
-0.379
|
|
(0.050)
|
(0.052)
|
|
p = 0.000***
|
p = 0.000***
|
|
|
|
GunFramexN
|
-1.425
|
-2.387
|
|
(1.154)
|
(1.218)
|
|
p = 0.218
|
p = 0.051*
|
|
|
|
GunFramexO
|
0.020
|
-1.077
|
|
(1.300)
|
(1.746)
|
|
p = 0.988
|
p = 0.538
|
|
|
|
GunFramexC
|
-1.268
|
-0.871
|
|
(1.446)
|
(1.516)
|
|
p = 0.382
|
p = 0.566
|
|
|
|
GunFramexE
|
0.878
|
0.937
|
|
(1.054)
|
(1.105)
|
|
p = 0.406
|
p = 0.398
|
|
|
|
GunFramexA
|
-3.306
|
-3.977
|
|
(1.373)
|
(1.454)
|
|
p = 0.017**
|
p = 0.007***
|
|
|
|
GunFramexPK
|
|
-1.175
|
|
|
(0.605)
|
|
|
p = 0.053*
|
|
|
|
GunFramexNC
|
|
0.748
|
|
|
(1.377)
|
|
|
p = 0.588
|
|
|
|
|
Observations
|
486
|
464
|
R2
|
0.171
|
0.175
|
Adjusted R2
|
0.155
|
0.155
|
Residual Std. Error
|
2.126 (df = 476)
|
2.122 (df = 452)
|
F Statistic
|
10.893*** (df = 9; 476)
|
8.740*** (df = 11; 452)
|
|
Note:
|
p<0.1; p<0.05; p<0.01
|